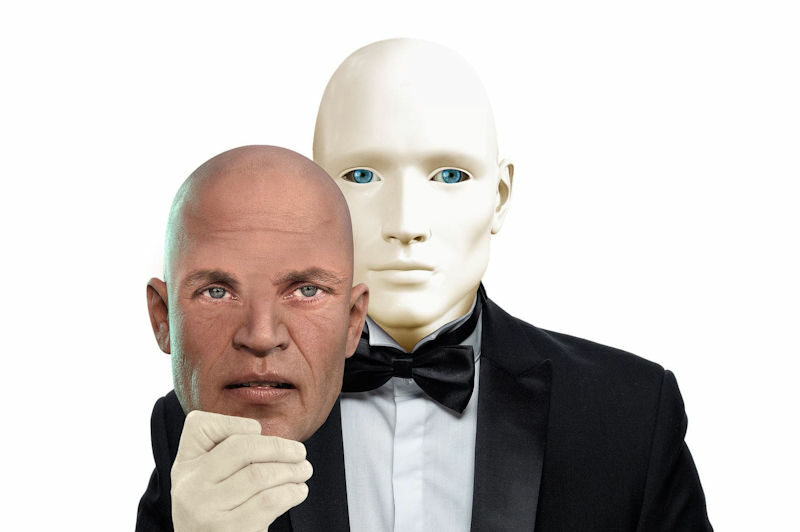
Synthetic image data extends machine learning capability
July 19, 2022The term “AI-enabled” is widely banded around software systems that claim to speed up machine learning. The reality is that AI platforms rely entirely on recognizing and learning from data and images that are pre-loaded, and “training” your system on these images still remains an important but laborious step in any AI transformation. At the end of the day, the system is only going to be as good as the data it first learnt from.
This means that training a system on as much information as possible is imperative, and the reliance on image data in particular is huge. Whether it’s teaching a vision system to identify cancer cells or instructing an autonomous vehicle on collision avoidance, good data can make life-changing differences.
Gathering images to “feed” machines can be both time-consuming and tricky, especially when privacy laws affect the collection of personal data and pictures. Companies are now cashing in on this increasing appetite for data by “creating” images for training. Synthetic data companies offer anything from computer-generated images of race-specific faces to help systems beat racism, to 3D renderings of the inner human body for interpreting anomalous cells.
Creating faces
Synthesis AI is one of many companies creating fake persona to be used in software training. Their data promises to encompass a range of environments and lighting situations, various poses and expressions, volumes of underrepresented groups and even near infrared (NI) images with their visual spectrum equivalents for models applied to NIR camera output.
As well as starting from scratch with facial datasets, it’s also possible to blend two or more real images together to create a new persona. One story explains how a beauty product provider needed to find images of women with facial hair but could only find a rich source of men in this category, photorealistic images were the answer!
Such software also hopes to address the underlying racist bias that’s been quoted as influencing training models since the inception of facial recognition. Claims are that too little training data from ethnic minorities and algorithms being developed typically by white males have led to computers being less accurate in identifying people of color. Indeed, error rates were found to be higher among black women than any other social group[1]. The introduction of synthetic data means that making a dataset more appropriate for a particular region or country should be simplified – training software will have access to reams of faces representing a wealth of different ages, sexes and races.
Synthetic data for medical imaging
Medical imaging machine learning programs also rely on a dataset rich in diversity and different scenarios. For example, skin lesions must be correctly identified and labelled regardless of skin color; the progression of tumors must be predicted as accurately as possible despite there being thousands of possible outcomes. Furthermore, collection of real-life data will be conducted under hugely dissimilar circumstances – some health settings use state-of-the-art imaging instruments, others in poorer or more remote locations may rely on a mobile phone. Contrast, scale, lighting and resolution of such images will be vastly different.
NVIDIA and King’s College London have collaborated to turn NVIDIA’s Cambridge-1 supercomputer into a generator for synthetic brains. King’s College researchers are building deep learning models which are able to synthesize artificial 3D MRI images of human brains. The C-1 supercomputer then enables accelerated generation of this synthetic data to build AI applications to better understand dementia, Parkinson’s and other brain disease.
Considering that AI is likely to be most useful in the identification and treatment of rare diseases, synthetic data offers a ground-breaking method of widening datasets without having to find real sufferers and obtain permission to use their data.
What are the risks?
Synthetic data appears to the be holy grail of AI platforms across a huge range of sectors. The advantage of this fake information is that there is apparent low risk of breaching privacy laws or facing data leaks – nobody can be endangered as nobody is real. Sound genius. But what are the risks?
Researchers have suggested that in some circumstances it’s possible to “roll back” fake data to find the real information underneath. This is especially problematic for image data created by merging facial features together. If a programmer wanted to, could they peel back the layers of the image to find one or more of the original photos and so expose the identity of the “faceless” faces? Some investigators believe this to be achievable.
In the case of medical data, if the underlying identity of an image associated with a particular disease can be discovered, this represents a serious breach of personal data. It’s a concern that synthetic data providers need to acknowledge and address. Furthermore, if an individual can use this method to discover that their image has been used to create data without their consent, lawsuits are likely to follow.
Going back to our earlier comment that AI can only be as good as the data it’s fed, quality of labeling is crucial. Savvy software companies are promoting labeling capabilities just as strongly as the breadth of their datasets. NVIDIA’s MONAI framework is an open-source platform comprised of labeling and learning tools that help researchers and clinicians collaborate, create annotated datasets easily and quickly, then connect them to NVIDIA’s Clara Holoscan platform for deployment. While this platform is designed specifically for medical imaging, we can expect other companies to produce similar offerings for other sectors.
Artificial data for artificial intelligence
Synthetic data undeniably delivers opportunities for machine learning to develop faster and wider than real-life records. As long as risks are understood and mitigated, the future of AI, and the associated industries employing it, are looking bright.
Read more about the use of AI in industry, healthcare and other sectors and follow us on social media for future updates.
[1] How is Face Recognition Surveillance Technology Racist? | News & Commentary | American Civil Liberties Union (aclu.org)